6. Exploring Advanced Features#
This notebook is target at advanced users that want, among other things, access MOA objects directly using the Python API from capymoa.
Examples on how to use any MOA Classifier or Regressor from capymoa
An example of how preprocessing (from MOA) can be used.
Comparing a SKLearn model against a MOA model
A variation of Tutorial 5:
Creating a new classifier in CapyMOA
which uses MOA learners, thus accessing MOA (Java) objects directlyHow to log experiments using TensorBoard alongside the PyTorch API. This extends Tutorial 3:
Using Pytorch with CapyMOA
Creating a synthetic stream with concept drifts using the MOA CLI directly
An example utilising a multi-threaded ensemble
More information about CapyMOA can be found in https://www.capymoa.org
last update on 28/07/2024
1. Using any MOA learner#
CapyMOA gives you access to any MOA classifier or regressor
For some of the MOA learners there are corresponding Python objects (such as the HoeffdingTree or Adaptive Random Forest Classifier). However, MOA has over a hundred learners, and more are added constantly.
To allow advanced users to access any MOA learner from CapyMOA, we included the
MOAClassifier
andMOARegressor
generic wrappers.
[1]:
from capymoa.evaluation import prequential_evaluation
from capymoa.base import MOAClassifier
from capymoa.datasets import RBFm_100k
# This is an import from MOA
from moa.classifiers.trees import HoeffdingAdaptiveTree
rbf_100k = RBFm_100k()
# Creates a wrapper around the HoeffdingAdaptiveTree, which then can be used as any other capymoa classifier
HAT = MOAClassifier(schema=rbf_100k.get_schema(), moa_learner=HoeffdingAdaptiveTree)
results_HAT = prequential_evaluation(stream=rbf_100k, learner=HAT, window_size=4500, max_instances=50000)
print(f"Cumulative accuracy = {results_HAT['cumulative'].accuracy()}, wall-clock time: {results_HAT['wallclock']}")
display(results_HAT['windowed'].metrics_per_window())
Cumulative accuracy = 59.57599999999999, wall-clock time: 1.4879562854766846
instances | accuracy | kappa | kappa_t | kappa_m | f1_score | f1_score_0 | f1_score_1 | f1_score_2 | f1_score_3 | ... | precision_1 | precision_2 | precision_3 | precision_4 | recall | recall_0 | recall_1 | recall_2 | recall_3 | recall_4 | |
---|---|---|---|---|---|---|---|---|---|---|---|---|---|---|---|---|---|---|---|---|---|
0 | 4500.0 | 50.000000 | 33.449507 | 34.858135 | 27.768860 | 45.089567 | 53.487412 | 33.012821 | 62.374245 | 13.945578 | ... | 39.845261 | 67.685590 | 29.496403 | 47.644540 | 43.338015 | 57.142857 | 28.180575 | 57.835821 | 9.131403 | 64.399421 |
1 | 9000.0 | 49.466667 | 32.401117 | 35.287422 | 28.332808 | 41.328952 | 56.127745 | 25.129983 | 61.844725 | 2.307692 | ... | 34.855769 | 68.228404 | 6.185567 | 45.596376 | 41.013784 | 59.175084 | 19.647696 | 56.553398 | 1.418440 | 68.274303 |
2 | 13500.0 | 52.422222 | 36.245962 | 38.846044 | 32.031746 | 45.928811 | 59.177456 | 25.333333 | 53.851590 | 18.657938 | ... | 39.820359 | 65.017065 | 31.318681 | 51.899384 | 43.756287 | 66.071429 | 18.575419 | 45.958987 | 13.286713 | 74.888889 |
3 | 18000.0 | 59.311111 | 46.626403 | 47.309353 | 41.632133 | 57.974404 | 59.917012 | 34.180139 | 58.620690 | 64.179104 | ... | 44.223108 | 56.342857 | 75.704225 | 64.530457 | 56.441207 | 62.946818 | 27.854454 | 61.090458 | 55.699482 | 74.614820 |
4 | 22500.0 | 64.555556 | 54.112305 | 54.258675 | 48.943662 | 63.436739 | 67.889126 | 52.967359 | 60.546875 | 63.816475 | ... | 62.962963 | 61.917443 | 57.954545 | 68.314763 | 63.573514 | 70.629991 | 45.710627 | 59.235669 | 70.997680 | 71.293605 |
5 | 27000.0 | 60.111111 | 48.455842 | 48.670289 | 44.375581 | 58.755137 | 68.893204 | 49.307075 | 58.830549 | 55.581395 | ... | 58.885017 | 56.536697 | 54.566210 | 60.080321 | 58.558489 | 73.671096 | 42.409034 | 61.318408 | 56.635071 | 58.758837 |
6 | 31500.0 | 60.666667 | 48.797681 | 49.225473 | 44.058154 | 59.622479 | 69.438669 | 47.246608 | 56.351039 | 61.557478 | ... | 56.813820 | 52.928416 | 66.223404 | 58.839590 | 58.587577 | 70.227082 | 40.437158 | 60.246914 | 57.505774 | 64.520958 |
7 | 36000.0 | 62.488889 | 51.365841 | 51.977240 | 46.666667 | 62.079191 | 67.826087 | 55.932203 | 53.044496 | 67.179487 | ... | 61.682243 | 50.727884 | 67.007673 | 65.527489 | 61.832537 | 68.997473 | 51.162791 | 55.582822 | 67.352185 | 66.067416 |
8 | 40500.0 | 57.933333 | 45.320224 | 45.852403 | 39.559387 | 56.433686 | 65.095729 | 35.967742 | 56.277603 | 61.290323 | ... | 43.984221 | 57.106274 | 59.507830 | 64.431725 | 56.395784 | 75.298126 | 30.422920 | 55.472637 | 63.182898 | 57.602339 |
9 | 45000.0 | 72.000000 | 63.510428 | 63.636364 | 59.420290 | 71.308445 | 71.458075 | 66.176471 | 72.941176 | 69.961977 | ... | 70.977918 | 71.480583 | 75.000000 | 74.647887 | 70.422343 | 74.121680 | 61.983471 | 74.462705 | 65.558195 | 75.985663 |
10 | 49500.0 | 66.177778 | 56.005059 | 55.820029 | 51.926721 | 66.012305 | 62.703583 | 61.891516 | 62.087186 | 70.573871 | ... | 65.058480 | 65.096953 | 71.534653 | 69.770674 | 65.432668 | 63.900415 | 59.018568 | 59.343434 | 69.638554 | 75.262369 |
11 | 50000.0 | 65.400000 | 54.995471 | 54.869565 | 50.712251 | 65.018349 | 61.386139 | 60.445682 | 61.479100 | 68.827930 | ... | 63.265306 | 63.479416 | 70.408163 | 70.110957 | 64.466325 | 62.155388 | 57.866667 | 59.600998 | 67.317073 | 75.391499 |
12 rows × 23 columns
1.1 Checking the hyperparameters for the MOA CLI#
MOA objects can be parametrized using the MOA CLI (Command Line Interface)
Sometimes you may not know the relevent parameters for
moa_learner
,moa_learner.CLI_help()
presents all the hyperparameters available for themoa_learner
object.
[2]:
from moa.classifiers.meta import AdaptiveRandomForest
arf = MOAClassifier(schema=rbf_100k.get_schema(), moa_learner=AdaptiveRandomForest)
print(arf.CLI_help())
-l treeLearner (default: ARFHoeffdingTree -e 2000000 -g 50 -c 0.01)
Random Forest Tree.
-s ensembleSize (default: 100)
The number of trees.
-o mFeaturesMode (default: Percentage (M * (m / 100)))
Defines how m, defined by mFeaturesPerTreeSize, is interpreted. M represents the total number of features.
-m mFeaturesPerTreeSize (default: 60)
Number of features allowed considered for each split. Negative values corresponds to M - m
-a lambda (default: 6.0)
The lambda parameter for bagging.
-j numberOfJobs (default: 1)
Total number of concurrent jobs used for processing (-1 = as much as possible, 0 = do not use multithreading)
-x driftDetectionMethod (default: ADWINChangeDetector -a 1.0E-3)
Change detector for drifts and its parameters
-p warningDetectionMethod (default: ADWINChangeDetector -a 1.0E-2)
Change detector for warnings (start training bkg learner)
-w disableWeightedVote
Should use weighted voting?
-u disableDriftDetection
Should use drift detection? If disabled then bkg learner is also disabled
-q disableBackgroundLearner
Should use bkg learner? If disabled then reset tree immediately.
2. Using preprocessing from MOA (filters)#
We are working on a more user friendly API for preprocessing, this example just show how one can do that using MOA filters from here
Here we use
NormalisationFilter
filter from MOA to normalize instances in an online fashion.MOA filters syntax wraps the whole stream, so we are always composing commands like `Filter(Stream,
We obtain the MOA CLI from the rbf_100k stream, since it can be mapped to a MOA stream, it is possible to obtain that. Comment out the print statements if you would like to inspect the actual creation strings (perhaps to copy and paste that into MOA?)
[3]:
from capymoa.stream import Stream
from capymoa.classifier import OnlineBagging
from capymoa.evaluation import prequential_evaluation
from moa.streams.filters import StandardisationFilter, NormalisationFilter
from moa.streams import FilteredStream
rbf_100k = RBFm_100k()
# print(f'MOA creation string for data: {rbf_100k.moa_stream.getCLICreationString(rbf_100k.moa_stream.__class__)}')
# Create a FilterStream and use the NormalisationFilter
rbf_stream_normalised = Stream(CLI=f"-s ({rbf_100k.moa_stream.getCLICreationString(rbf_100k.moa_stream.__class__)}) \
-f NormalisationFilter ", moa_stream=FilteredStream())
# print(f'MOA creation string for filtered version: {rbf_stream_normalised.moa_stream.getCLICreationString(rbf_stream_normalised.moa_stream.__class__)}')
ob_learner_norm = OnlineBagging(schema=rbf_stream_normalised.get_schema(), ensemble_size=5)
ob_learner = OnlineBagging(schema=rbf_100k.get_schema(), ensemble_size=5)
ob_results_norm = prequential_evaluation(stream=rbf_stream_normalised, learner=ob_learner_norm)
ob_results = prequential_evaluation(stream=rbf_100k, learner=ob_learner)
print(f"Accuracy with online normalization: {ob_results_norm['cumulative'].accuracy()}")
print(f"Accuracy without normalization: {ob_results['cumulative'].accuracy()}")
Accuracy with online normalization: 61.815
Accuracy without normalization: 60.357000000000006
3. Comparing a MOA and SKLearn models#
This simple example shows how it is simple to compare a MOA and a SKLearn regressors.
For the sake of this example, we are using the wrappers
SKClassifier (and SKRegressor) are parametrized directly as part of the object initialization
MOAClassifier (and MOARegressor) are parametrized through a CLI (a separate parameter)
[4]:
from capymoa.base import SKClassifier, MOAClassifier
from capymoa.datasets import CovtypeTiny
from capymoa.evaluation import prequential_evaluation_multiple_learners
from capymoa.evaluation.visualization import plot_windowed_results
from sklearn.linear_model import SGDClassifier
from moa.classifiers.trees import HoeffdingTree
covt_tiny = CovtypeTiny()
sk_sgd = SKClassifier(schema=covt_tiny.schema, sklearner=SGDClassifier(loss='log_loss', penalty='l1', alpha=0.001))
moa_ht = MOAClassifier(schema=covt_tiny.schema, moa_learner=HoeffdingTree, CLI="-g 50")
results = prequential_evaluation_multiple_learners(stream=covt_tiny, learners={'sk_sgd':sk_sgd, 'moa_ht':moa_ht}, window_size=100)
plot_windowed_results(results['sk_sgd'], results['moa_ht'], metric='accuracy')
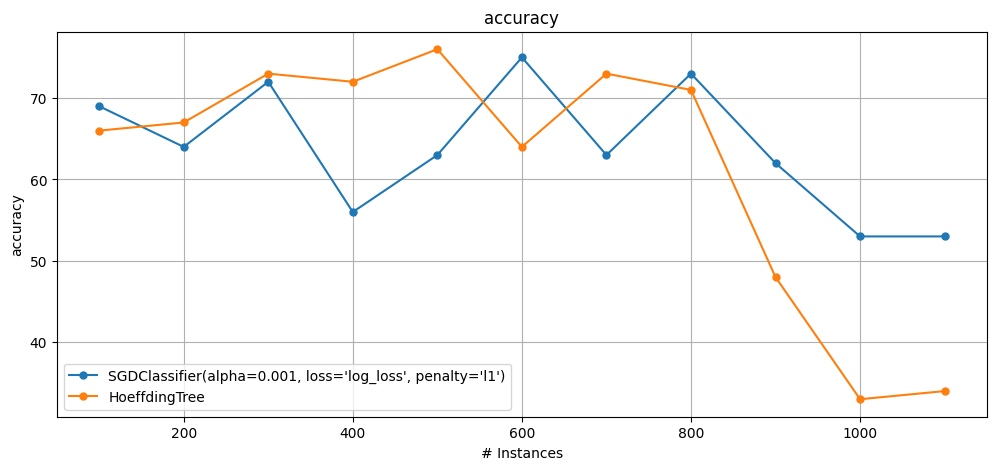
4. Creating Python learners with MOA Objects#
This example follow the example from
06_new_learner
which shows how to create a custom online bagging implementation.Here we also create an online bagging implementation, but the base_learner is a MOA class
[5]:
from capymoa.base import Classifier, MOAClassifier
from moa.classifiers.trees import HoeffdingTree
from collections import Counter
import numpy as np
import random
import math
def poisson(lambd, random_generator):
if lambd < 100.0:
product = 1.0
_sum = 1.0
threshold = random_generator.random() * math.exp(lambd)
i = 1
max_val = max(100, 10 * math.ceil(lambd))
while i < max_val and _sum <= threshold:
product *= (lambd / i)
_sum += product
i += 1
return i - 1
x = lambd + math.sqrt(lambd) * random_generator.gauss(0, 1)
if x < 0.0:
return 0
return int(math.floor(x))
class CustomOnlineBagging(Classifier):
def __init__(self, schema=None, random_seed=1, ensemble_size=5, moa_base_learner_class=None, CLI_base_learner=None):
super().__init__(schema=schema, random_seed=random_seed)
self.random_generator = random.Random()
self.CLI_base_learner = CLI_base_learner
self.ensemble_size = ensemble_size
self.moa_base_learner_class = moa_base_learner_class
# Default base learner if None is specified
if self.moa_base_learner_class is None:
self.moa_base_learner_class = HoeffdingTree
self.ensemble = []
# Create several instances for the base_learners
for i in range(self.ensemble_size):
self.ensemble.append(MOAClassifier(schema=self.schema, moa_learner=self.moa_base_learner_class(), CLI=self.CLI_base_learner))
def __str__(self):
return 'CustomOnlineBagging'
def train(self, instance):
for i in range(self.ensemble_size):
k = poisson(1.0, self.random_generator)
for _ in range(k):
self.ensemble[i].train(instance)
def predict(self, instance):
predictions = []
for i in range(self.ensemble_size):
predictions.append(self.ensemble[i].predict(instance))
majority_vote = Counter(predictions)
prediction = majority_vote.most_common(1)[0][0]
return prediction
def predict_proba(self, instance):
probabilities = []
for i in range(self.ensemble_size):
classifier_proba = self.ensemble[i].predict_proba(instance)
classifier_proba = classifier_proba / np.sum(classifier_proba)
probabilities.append(classifier_proba)
avg_proba = np.mean(probabilities, axis=0)
return avg_proba
4.1 Testing the custom online bagging#
We choose to use an HoeffdingAdaptiveTree from MOA as the base learner
We also specify the CLI commands to configure the base learner
[6]:
from capymoa.evaluation import prequential_evaluation
from capymoa.stream import stream_from_file
from moa.classifiers.trees import HoeffdingAdaptiveTree
elec_stream = stream_from_file(path_to_csv_or_arff="../data/electricity.csv")
# Creating a learner: using a hoeffding adaptive tree as the base learner with grace period of 50 (-g 50)
NEW_OB = CustomOnlineBagging(schema=elec_stream.get_schema(),
ensemble_size=5,
moa_base_learner_class=HoeffdingAdaptiveTree,
CLI_base_learner="-g 50")
results_NEW_OB = prequential_evaluation(stream=elec_stream, learner=NEW_OB, window_size=4500)
print(f"Accuracy: {results_NEW_OB.cumulative.accuracy()}"
/Users/gomeshe/Dropbox/ciencia_computacao/dev/main-projects/CapyMOA/src/capymoa/stream/_stream.py:38: UserWarning: target variable includes 2 (< 20) unique values, inferred as categorical, set target_type = 'numeric' if you intend numeric targets
warnings.warn(f'target variable includes {num_unique} (< 20) unique values, inferred as categorical, '
[6]:
82.58077330508475
5. Using TensorBoard with PyTorch in CapyMOA#
One can use TensorBoard to visualize logged data in an online fashion
We go through all the steps below, including installing TensorBoard
5.1 Install TensorBoard#
Clear any logs from previous runs
rm -rf ./runs
[7]:
!pip install tensorboard
Requirement already satisfied: tensorboard in /Users/gomeshe/miniconda3/envs/capymoa/lib/python3.9/site-packages (2.17.0)
Requirement already satisfied: absl-py>=0.4 in /Users/gomeshe/miniconda3/envs/capymoa/lib/python3.9/site-packages (from tensorboard) (2.1.0)
Requirement already satisfied: grpcio>=1.48.2 in /Users/gomeshe/miniconda3/envs/capymoa/lib/python3.9/site-packages (from tensorboard) (1.65.1)
Requirement already satisfied: markdown>=2.6.8 in /Users/gomeshe/miniconda3/envs/capymoa/lib/python3.9/site-packages (from tensorboard) (3.6)
Requirement already satisfied: numpy>=1.12.0 in /Users/gomeshe/miniconda3/envs/capymoa/lib/python3.9/site-packages (from tensorboard) (1.26.3)
Requirement already satisfied: protobuf!=4.24.0,<5.0.0,>=3.19.6 in /Users/gomeshe/miniconda3/envs/capymoa/lib/python3.9/site-packages (from tensorboard) (4.25.4)
Requirement already satisfied: setuptools>=41.0.0 in /Users/gomeshe/miniconda3/envs/capymoa/lib/python3.9/site-packages (from tensorboard) (69.5.1)
Requirement already satisfied: six>1.9 in /Users/gomeshe/miniconda3/envs/capymoa/lib/python3.9/site-packages (from tensorboard) (1.16.0)
Requirement already satisfied: tensorboard-data-server<0.8.0,>=0.7.0 in /Users/gomeshe/miniconda3/envs/capymoa/lib/python3.9/site-packages (from tensorboard) (0.7.2)
Requirement already satisfied: werkzeug>=1.0.1 in /Users/gomeshe/miniconda3/envs/capymoa/lib/python3.9/site-packages (from tensorboard) (3.0.3)
Requirement already satisfied: importlib-metadata>=4.4 in /Users/gomeshe/miniconda3/envs/capymoa/lib/python3.9/site-packages (from markdown>=2.6.8->tensorboard) (7.1.0)
Requirement already satisfied: MarkupSafe>=2.1.1 in /Users/gomeshe/miniconda3/envs/capymoa/lib/python3.9/site-packages (from werkzeug>=1.0.1->tensorboard) (2.1.5)
Requirement already satisfied: zipp>=0.5 in /Users/gomeshe/miniconda3/envs/capymoa/lib/python3.9/site-packages (from importlib-metadata>=4.4->markdown>=2.6.8->tensorboard) (3.18.2)
5.2 PyTorchClassifier#
We define
PyTorchClassifier
andNeuralNetwork
classes similarly to those from Tutorial 3:Using Pytorch with CapyMOA
[8]:
from capymoa.base import Classifier
import numpy as np
import torch
from torch import nn
torch.manual_seed(1)
torch.use_deterministic_algorithms(True)
# Get cpu device for training.
device = ("cpu")
# Define model
class NeuralNetwork(nn.Module):
def __init__(self, input_size=0, number_of_classes=0):
super().__init__()
self.flatten = nn.Flatten()
self.linear_relu_stack = nn.Sequential(
nn.Linear(input_size, 512),
nn.ReLU(),
nn.Linear(512, 512),
nn.ReLU(),
nn.Linear(512, number_of_classes)
)
def forward(self, x):
x = self.flatten(x)
logits = self.linear_relu_stack(x)
return logits
class PyTorchClassifier(Classifier):
def __init__(self, schema=None, random_seed=1, nn_model: nn.Module = None, optimizer=None, loss_fn=nn.CrossEntropyLoss(), device=("cpu"), lr=1e-3):
super().__init__(schema, random_seed)
self.model = None
self.optimizer = None
self.loss_fn = loss_fn
self.lr = lr
self.device = device
torch.manual_seed(random_seed)
if nn_model is None:
self.set_model(None)
else:
self.model = nn_model.to(device)
if optimizer is None:
if self.model is not None:
self.optimizer = torch.optim.SGD(self.model.parameters(), lr=lr)
else:
self.optimizer = optimizer
def __str__(self):
return str(self.model)
def CLI_help(self):
return str('schema=None, random_seed=1, nn_model: nn.Module = None, optimizer=None, loss_fn=nn.CrossEntropyLoss(), device=("cpu"), lr=1e-3')
def set_model(self, instance):
if self.schema is None:
moa_instance = instance.java_instance.getData()
self.model = NeuralNetwork(input_size=moa_instance.get_num_attributes(), number_of_classes=moa_instance.get_num_classes()).to(self.device)
elif instance is not None:
self.model = NeuralNetwork(input_size=self.schema.get_num_attributes(), number_of_classes=self.schema.get_num_classes()).to(self.device)
def train(self, instance):
if self.model is None:
self.set_model(instance)
X = torch.tensor(instance.x, dtype=torch.float32)
y = torch.tensor(instance.y_index, dtype=torch.long)
# set the device and add a dimension to the tensor
X, y = torch.unsqueeze(X.to(self.device), 0), torch.unsqueeze(y.to(self.device),0)
# Compute prediction error
pred = self.model(X)
loss = self.loss_fn(pred, y)
# Backpropagation
loss.backward()
self.optimizer.step()
self.optimizer.zero_grad()
def predict(self, instance):
return np.argmax(self.predict_proba(instance))
def predict_proba(self, instance):
if self.model is None:
self.set_model(instance)
X = torch.unsqueeze(torch.tensor(instance.x, dtype=torch.float32).to(self.device), 0)
# turn off gradient collection
with torch.no_grad():
pred = np.asarray(self.model(X).numpy(), dtype=np.double)
return pred
5.3 PyTorchClassifier + the test-then-train loop + TensorBoard#
Here we use instance loop to log relevant log information to TensorBoard
These information can be viewed while the processing is happening using TensorBoard
[9]:
from capymoa.evaluation import ClassificationEvaluator
from capymoa.datasets import RBFm_100k
from torch.utils.tensorboard import SummaryWriter
# Create a SummaryWriter instance.
writer = SummaryWriter()
## Opening a file again to start from the beginning
rbf_stream = RBFm_100k()
# Creating the evaluator
evaluator = ClassificationEvaluator(schema=rbf_stream.get_schema())
# Creating a learner
simple_pyTorch_classifier = PyTorchClassifier(
schema=rbf_stream.get_schema(),
nn_model=NeuralNetwork(input_size=rbf_stream.get_schema().get_num_attributes(),
number_of_classes=rbf_stream.get_schema().get_num_classes()).to(device)
)
i = 0
while rbf_stream.has_more_instances():
i += 1
instance = rbf_stream.next_instance()
prediction = simple_pyTorch_classifier.predict(instance)
evaluator.update(instance.y_index, prediction)
simple_pyTorch_classifier.train(instance)
if i % 1000 == 0:
writer.add_scalar("accuracy", evaluator.accuracy(), i)
if i % 10000 == 0:
print(f"Processed {i} instances")
writer.add_scalar("accuracy", evaluator.accuracy(), i)
# Call flush() method to make sure that all pending events have been written to disk.
writer.flush()
# If you do not need the summary writer anymore, call close() method.
writer.close()
Processed 10000 instances
Processed 20000 instances
Processed 30000 instances
Processed 40000 instances
Processed 50000 instances
Processed 60000 instances
Processed 70000 instances
Processed 80000 instances
Processed 90000 instances
Processed 100000 instances
5.4 Run TensorBoard#
Now, start TensorBoard, specifying the root log directory you used above. Argument logdir
points to directory where TensorBoard will look to find event files that it can display. TensorBoard will recursively walk the directory structure rooted at logdir
, looking for .*tfevents.*
files.
tensorboard --logdir=runs
Go to the URL it provides
This dashboard shows how the accuracy change with time. You can use it to also track training speed, learning rate, and other scalar values.
6. Creating a synthetic stream with concept drifts from MOA#
Demonstrates the flexibility of the API, these level of manipulation of the API is expected from experienced MOA users.
To use the API like this the user must be familiar with how concept drifts are simulatd in MOA
EvaluatePrequential -l trees.HoeffdingAdaptiveTree -s (ConceptDriftStream -s generators.AgrawalGenerator -d (generators.AgrawalGenerator -f 2) -p 5000) -e (WindowClassificationPerformanceEvaluator -w 100) -i 10000 -f 100
[10]:
from capymoa.stream import Stream
from capymoa.classifier import OnlineBagging
from capymoa.evaluation import prequential_evaluation
from capymoa.evaluation.visualization import plot_windowed_results
from moa.streams import ConceptDriftStream
# Using the API to generate the data using the ConceptDriftStream and AgrawalGenerator.
# The drift location is based on the number of instances (5000) as well as the drift width (1000, the default value)
stream_sea1drift = Stream(moa_stream=ConceptDriftStream(),
CLI="-s generators.SEAGenerator -d (generators.SEAGenerator -f 2) -p 5000 -w 1000")
OB = OnlineBagging(schema=stream_sea1drift.get_schema(), ensemble_size=10)
results_sea1drift_OB = prequential_evaluation(stream=stream_sea1drift, learner=OB, window_size=100, max_instances=10000)
plot_windowed_results(results_sea1drift_OB, metric='accuracy')
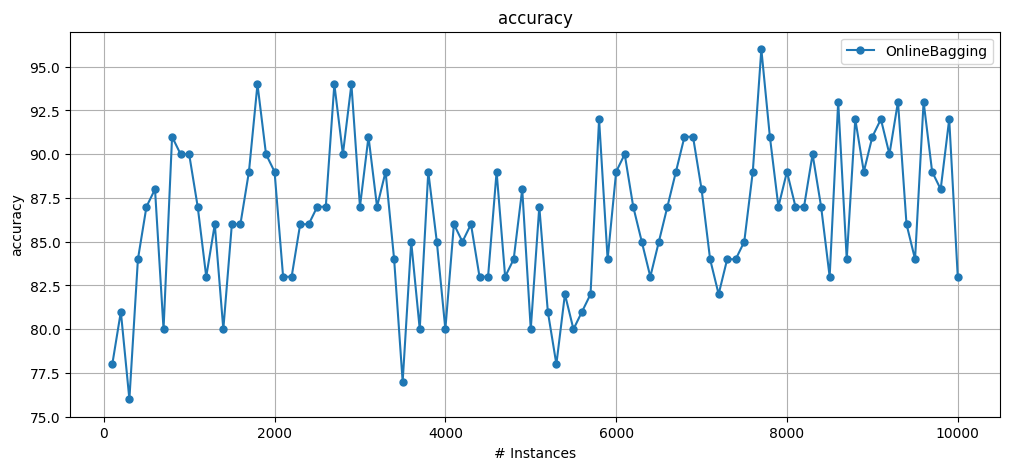
7. Drift, Multi-threated Ensemble and Results#
Generate a stream with 3 drifts, 2 abrupt and one gradual.
Evaluate utilising test-then-train (cumulative) and windowed evaluation.
Execute a multi-threated version of AdaptiveRandomForest.
For more on multi-threaded ensembles, see parallel_ensembles.ipynb notebook
[12]:
from capymoa.stream.generator import SEA
from capymoa.stream.drift import DriftStream, AbruptDrift, GradualDrift
from capymoa.classifier import AdaptiveRandomForestClassifier
from capymoa.evaluation import prequential_evaluation
from capymoa.evaluation.visualization import plot_windowed_results
SEA3drifts = DriftStream(stream=[SEA(1),
AbruptDrift(10000),
SEA(2),
GradualDrift(start=20000, end=25000),
SEA(3),
AbruptDrift(45000),
SEA(1)])
arf = AdaptiveRandomForestClassifier(schema=SEA3drifts.get_schema(),
ensemble_size=100,
number_of_jobs=4)
results = prequential_evaluation(stream=SEA3drifts,
learner=arf,
window_size=5000,
max_instances=50000)
print(f"Cumulative accuracy = {results.cumulative.accuracy()}")
print(f"wallclock = {results.wallclock()} seconds")
display(results.windowed.metrics_per_window())
plot_windowed_results(results, metric='accuracy')
None
Cumulative accuracy = 89.37
wallclock = 14.674108982086182 seconds
instances | accuracy | kappa | kappa_t | kappa_m | f1_score | f1_score_0 | f1_score_1 | precision | precision_0 | precision_1 | recall | recall_0 | recall_1 | |
---|---|---|---|---|---|---|---|---|---|---|---|---|---|---|
0 | 5000.0 | 88.26 | 73.743687 | 74.333188 | 67.096413 | 87.033480 | 82.534960 | 91.158307 | 88.176897 | 87.951807 | 88.401987 | 85.919338 | 77.746637 | 94.092040 |
1 | 10000.0 | 88.88 | 75.482652 | 76.520270 | 69.700272 | 87.903177 | 83.939919 | 91.495870 | 88.990120 | 89.305470 | 88.674770 | 86.842465 | 79.182561 | 94.502370 |
2 | 15000.0 | 89.26 | 76.181571 | 77.187766 | 70.315091 | 88.238991 | 84.302835 | 91.837665 | 89.310872 | 89.454094 | 89.167651 | 87.192532 | 79.712548 | 94.672516 |
3 | 20000.0 | 88.98 | 75.124468 | 75.938865 | 68.586089 | 87.733308 | 83.297969 | 91.777347 | 88.966743 | 88.932039 | 89.001447 | 86.533606 | 78.335234 | 94.731978 |
4 | 25000.0 | 89.90 | 77.706053 | 78.157439 | 72.114854 | 88.955206 | 85.400405 | 92.279468 | 89.829793 | 89.623786 | 90.035800 | 88.097484 | 81.557151 | 94.637817 |
5 | 30000.0 | 89.50 | 76.471976 | 76.933216 | 70.522179 | 88.417299 | 84.314311 | 92.108823 | 89.663782 | 90.102171 | 89.225393 | 87.204997 | 79.225154 | 95.184840 |
6 | 35000.0 | 90.10 | 77.634753 | 78.232190 | 71.535365 | 88.949725 | 84.986351 | 92.615247 | 90.051553 | 89.922978 | 90.180128 | 87.874534 | 80.563542 | 95.185526 |
7 | 40000.0 | 89.70 | 77.159091 | 77.676636 | 71.325167 | 88.687154 | 84.963504 | 92.167300 | 89.601454 | 89.318600 | 89.884307 | 87.791326 | 81.013363 | 94.569288 |
8 | 45000.0 | 89.58 | 76.658626 | 77.406765 | 70.531674 | 88.460627 | 84.461676 | 92.161878 | 89.515036 | 89.337539 | 89.692533 | 87.430769 | 80.090498 | 94.771040 |
9 | 50000.0 | 89.54 | 77.044005 | 77.697228 | 71.637744 | 88.656788 | 85.052872 | 91.955084 | 89.633308 | 89.909366 | 89.357250 | 87.701317 | 80.694143 | 94.708492 |
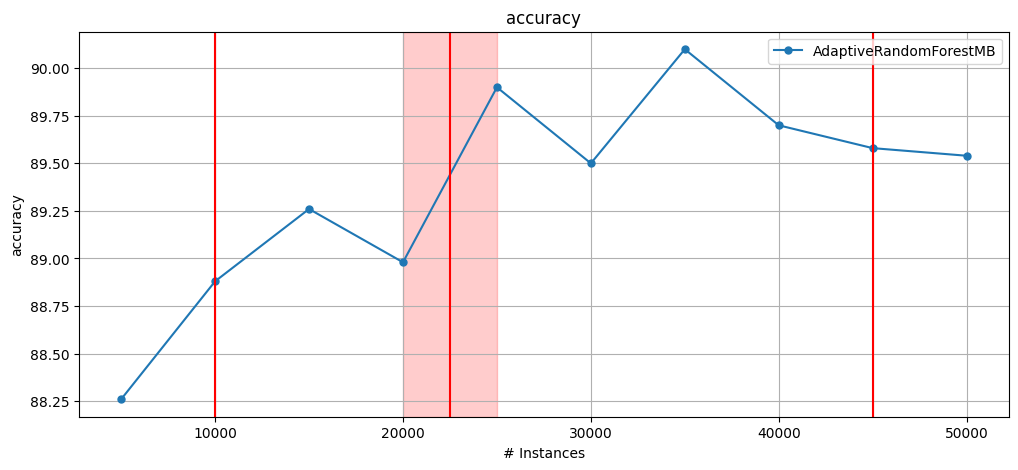
[ ]: