Anomaly Detection#
This notebook shows some basic usage of CapyMOA for Anomaly Detection tasks.
Algorithms: HalfSpaceTrees
, Autoencoder
and Online Isolation Forest
Important notes: Prior to version 0.8.2, a lower anomaly score indicated a higher likelihood of an anomaly. This has been updated so that a higher anomaly score now indicates a higher likelihood of an anomaly, aligning with the standard anomaly detection literature.
More information about CapyMOA can be found in https://www.capymoa.org
last update on 10/01/2025
1. Creating simple anomalous data with sklearn
#
Generating a few examples and some simple anomalous data using
sklearn
[1]:
import numpy as np
from sklearn.datasets import make_blobs
import matplotlib.pyplot as plt
from capymoa.stream import NumpyStream
# generate normal data points
n_samples = 10000
n_features = 2
n_clusters = 3
X, y = make_blobs(
n_samples=n_samples, n_features=n_features, centers=n_clusters, random_state=42
)
# generate anomalous data points
n_anomalies = 100 # the anomaly rate is 1%
anomalies = np.random.uniform(low=-10, high=10, size=(n_anomalies, n_features))
# combine the normal data points with anomalies
X = np.vstack([X, anomalies])
y = np.hstack([y, [1] * n_anomalies]) # Label anomalies with 1
y[:n_samples] = 0 # Label normal points with 0
# shuffle the data
idx = np.random.permutation(n_samples + n_anomalies)
X = X[idx]
y = y[idx]
plt.scatter(X[:, 0], X[:, 1], c=y, cmap="viridis")
plt.show()
# create a NumpyStream from the combined dataset
feature_names = [f"feature_{i}" for i in range(n_features)]
target_name = "class"
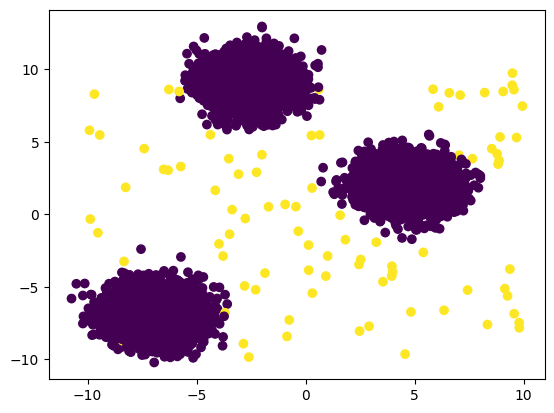
2. Unsupervised Anomaly Detection for data streams#
Recent research has been focused on unsupervised anomaly detection for data streams, as it is often difficult to obtain labeled data for training.
Instead of using evaluation functions, we first use a basic test-then-train loop from scratch to evaluate the model’s performance.
Please notice that higher scores indicate higher anomaly likelihood.
[2]:
from capymoa.anomaly import HalfSpaceTrees
from capymoa.evaluation import AnomalyDetectionEvaluator
stream_ad = NumpyStream(
X,
y,
dataset_name="AnomalyDetectionDataset",
feature_names=feature_names,
target_name=target_name,
target_type="categorical",
)
learner = HalfSpaceTrees(stream_ad.get_schema())
evaluator = AnomalyDetectionEvaluator(stream_ad.get_schema())
while stream_ad.has_more_instances():
instance = stream_ad.next_instance()
score = learner.score_instance(instance)
evaluator.update(instance.y_index, score)
learner.train(instance)
auc = evaluator.auc()
print(f"AUC: {auc:.2f}")
AUC: 0.94
3. High-level evaluation functions#
CapyMOA provides
prequential_evaluation_anomaly
as a high level function to assess Anomaly Detectors
3.1 prequential_evaluation_anomaly
#
In this example, we use the prequential_evaluation_anomaly
function with plot_windowed_results
to plot AUC for HalfSpaceTrees on the synthetic data stream
[3]:
from capymoa.evaluation.visualization import plot_windowed_results
from capymoa.anomaly import HalfSpaceTrees
from capymoa.evaluation import prequential_evaluation_anomaly
stream_ad = NumpyStream(
X,
y,
dataset_name="AnomalyDetectionDataset",
feature_names=feature_names,
target_name=target_name,
target_type="categorical",
)
hst = HalfSpaceTrees(schema=stream_ad.get_schema())
results_hst = prequential_evaluation_anomaly(
stream=stream_ad, learner=hst, window_size=1000
)
print(f"AUC: {results_hst.auc()}")
display(results_hst.windowed.metrics_per_window())
plot_windowed_results(results_hst, metric="auc", save_only=False)
AUC: 0.9415815
instances | auc | s_auc | Accuracy | Kappa | Periodical holdout AUC | Pos/Neg ratio | G-Mean | Recall | KappaM | |
---|---|---|---|---|---|---|---|---|---|---|
0 | 1000.0 | 0.849040 | 0.180372 | 0.060 | -0.001022 | 0.000000 | 0.010101 | 0.215322 | 0.9 | -93.000000 |
1 | 2000.0 | 0.945619 | 0.196099 | 0.007 | 0.000000 | 0.849040 | 0.007049 | 0.000000 | 1.0 | -115.823529 |
2 | 3000.0 | 0.965010 | 0.215852 | 0.014 | 0.000000 | 0.945619 | 0.014199 | 0.000000 | 1.0 | -94.419355 |
3 | 4000.0 | 0.927062 | 0.200816 | 0.006 | 0.000000 | 0.965010 | 0.006036 | 0.000000 | 1.0 | -106.459459 |
4 | 5000.0 | 0.929814 | 0.185818 | 0.008 | 0.000000 | 0.927062 | 0.008065 | 0.000000 | 1.0 | -109.222222 |
5 | 6000.0 | 0.944977 | 0.206376 | 0.013 | 0.000000 | 0.929814 | 0.013171 | 0.000000 | 1.0 | -101.103448 |
6 | 7000.0 | 0.955576 | 0.207342 | 0.013 | 0.000000 | 0.944977 | 0.013171 | 0.000000 | 1.0 | -96.309859 |
7 | 8000.0 | 0.972828 | 0.221292 | 0.010 | 0.000000 | 0.955576 | 0.010101 | 0.000000 | 1.0 | -96.777778 |
8 | 9000.0 | 0.937626 | 0.216386 | 0.008 | 0.000000 | 0.972828 | 0.008065 | 0.000000 | 1.0 | -99.314607 |
9 | 10000.0 | 0.976118 | 0.219181 | 0.009 | 0.000000 | 0.937626 | 0.009082 | 0.000000 | 1.0 | -100.122449 |
10 | 10100.0 | 0.977778 | 0.222641 | 0.010 | 0.000000 | 0.976118 | 0.010101 | 0.000000 | 1.0 | -98.990000 |
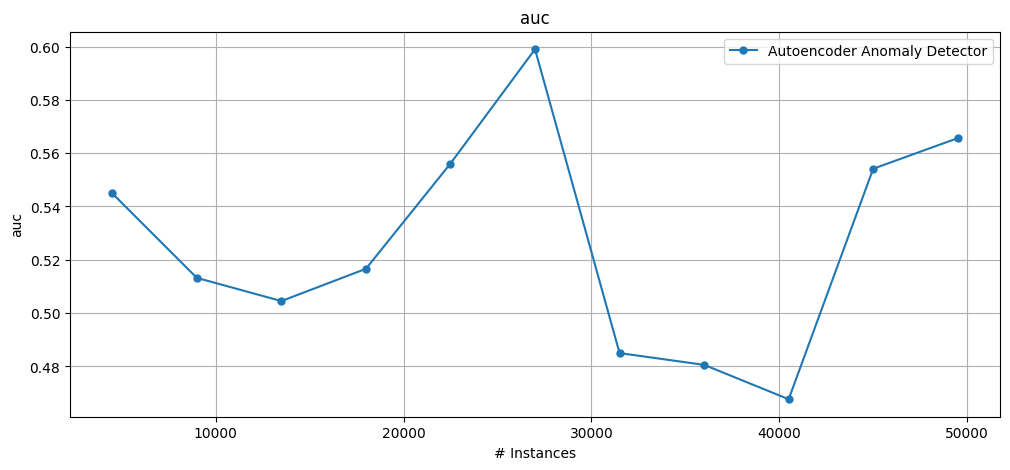
3.2 Autoencoder#
[4]:
from capymoa.evaluation.visualization import plot_windowed_results
from capymoa.anomaly import Autoencoder
from capymoa.evaluation import prequential_evaluation_anomaly
stream_ad = NumpyStream(
X,
y,
dataset_name="AnomalyDetectionDataset",
feature_names=feature_names,
target_name=target_name,
target_type="categorical",
)
ae = Autoencoder(schema=stream_ad.get_schema(), hidden_layer=1)
results_ae = prequential_evaluation_anomaly(
stream=stream_ad, learner=ae, window_size=1000
)
print(f"AUC: {results_ae.auc()}")
display(results_ae.windowed.metrics_per_window())
plot_windowed_results(results_ae, metric="auc", save_only=False)
AUC: 0.54006
instances | auc | s_auc | Accuracy | Kappa | Periodical holdout AUC | Pos/Neg ratio | G-Mean | Recall | KappaM | |
---|---|---|---|---|---|---|---|---|---|---|
0 | 1000.0 | 0.477778 | 0.015538 | 0.989 | -0.001821 | 0.000000 | 0.010101 | 0.000000 | 0.0 | -0.100000 |
1 | 2000.0 | 0.545533 | 0.028975 | 0.993 | 0.000000 | 0.477778 | 0.007049 | 0.000000 | 0.0 | 0.176471 |
2 | 3000.0 | 0.557664 | 0.000238 | 0.986 | 0.000000 | 0.545533 | 0.014199 | 0.000000 | 0.0 | -0.354839 |
3 | 4000.0 | 0.615023 | 0.000008 | 0.994 | 0.000000 | 0.557664 | 0.006036 | 0.000000 | 0.0 | 0.351351 |
4 | 5000.0 | 0.523438 | 0.000445 | 0.991 | -0.001781 | 0.615023 | 0.008065 | 0.000000 | 0.0 | 0.000000 |
5 | 6000.0 | 0.428727 | 0.009665 | 0.986 | -0.001861 | 0.523438 | 0.013171 | 0.000000 | 0.0 | -0.448276 |
6 | 7000.0 | 0.545554 | 0.000071 | 0.986 | -0.001861 | 0.428727 | 0.013171 | 0.000000 | 0.0 | -0.380282 |
7 | 8000.0 | 0.670808 | 0.061258 | 0.991 | 0.180328 | 0.545554 | 0.010101 | 0.316228 | 0.1 | 0.111111 |
8 | 9000.0 | 0.597908 | 0.042223 | 0.991 | -0.001781 | 0.670808 | 0.008065 | 0.000000 | 0.0 | 0.089888 |
9 | 10000.0 | 0.588070 | 0.041752 | 0.991 | 0.000000 | 0.597908 | 0.009082 | 0.000000 | 0.0 | 0.081633 |
10 | 10100.0 | 0.553131 | 0.037586 | 0.990 | 0.000000 | 0.588070 | 0.010101 | 0.000000 | 0.0 | -0.010000 |
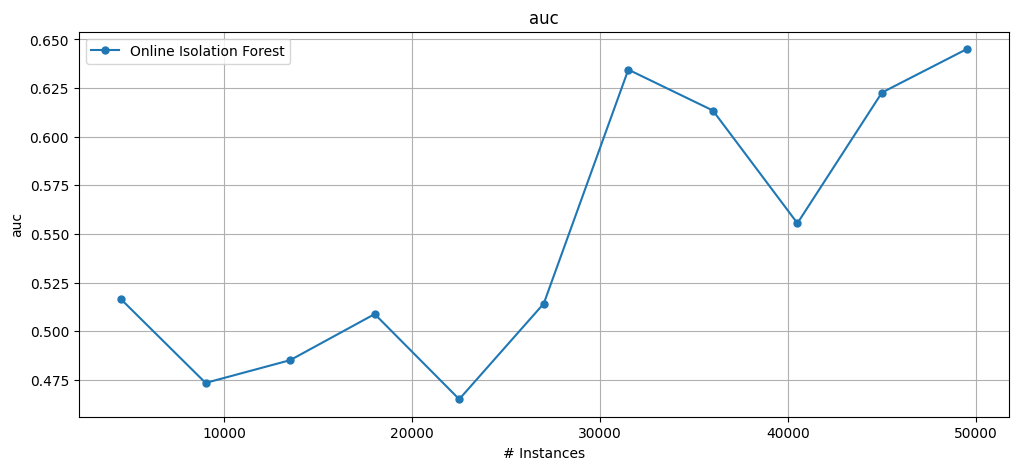
3.3 Online Isolation Forest#
[5]:
from capymoa.evaluation.visualization import plot_windowed_results
from capymoa.anomaly import OnlineIsolationForest
from capymoa.evaluation import prequential_evaluation_anomaly
stream_ad = NumpyStream(
X,
y,
dataset_name="AnomalyDetectionDataset",
feature_names=feature_names,
target_name=target_name,
target_type="categorical",
)
oif = OnlineIsolationForest(schema=stream_ad.get_schema(), num_trees=10)
results_oif = prequential_evaluation_anomaly(
stream=stream_ad, learner=oif, window_size=1000
)
print(f"AUC: {results_oif.auc()}")
display(results_oif.windowed.metrics_per_window())
plot_windowed_results(results_oif, metric="auc", save_only=False)
AUC: 0.643632
instances | auc | s_auc | Accuracy | Kappa | Periodical holdout AUC | Pos/Neg ratio | G-Mean | Recall | KappaM | |
---|---|---|---|---|---|---|---|---|---|---|
0 | 1000.0 | 0.609091 | 0.111064 | 0.959 | 0.031649 | 0.000000 | 0.010101 | 0.311075 | 0.1 | -3.100000 |
1 | 2000.0 | 0.767228 | 0.063018 | 0.993 | 0.000000 | 0.609091 | 0.007049 | 0.000000 | 0.0 | 0.176471 |
2 | 3000.0 | 0.678463 | 0.060250 | 0.986 | 0.000000 | 0.767228 | 0.014199 | 0.000000 | 0.0 | -0.354839 |
3 | 4000.0 | 0.680835 | 0.083064 | 0.994 | 0.000000 | 0.678463 | 0.006036 | 0.000000 | 0.0 | 0.351351 |
4 | 5000.0 | 0.632560 | 0.057094 | 0.992 | 0.000000 | 0.680835 | 0.008065 | 0.000000 | 0.0 | 0.111111 |
5 | 6000.0 | 0.632492 | 0.085781 | 0.987 | 0.000000 | 0.632560 | 0.013171 | 0.000000 | 0.0 | -0.344828 |
6 | 7000.0 | 0.585457 | 0.043215 | 0.987 | 0.000000 | 0.632492 | 0.013171 | 0.000000 | 0.0 | -0.281690 |
7 | 8000.0 | 0.789848 | 0.082476 | 0.990 | 0.000000 | 0.585457 | 0.010101 | 0.000000 | 0.0 | 0.012346 |
8 | 9000.0 | 0.675025 | 0.069445 | 0.992 | 0.000000 | 0.789848 | 0.008065 | 0.000000 | 0.0 | 0.191011 |
9 | 10000.0 | 0.649849 | 0.066430 | 0.991 | 0.000000 | 0.675025 | 0.009082 | 0.000000 | 0.0 | 0.081633 |
10 | 10100.0 | 0.613182 | 0.059306 | 0.990 | 0.000000 | 0.649849 | 0.010101 | 0.000000 | 0.0 | -0.010000 |
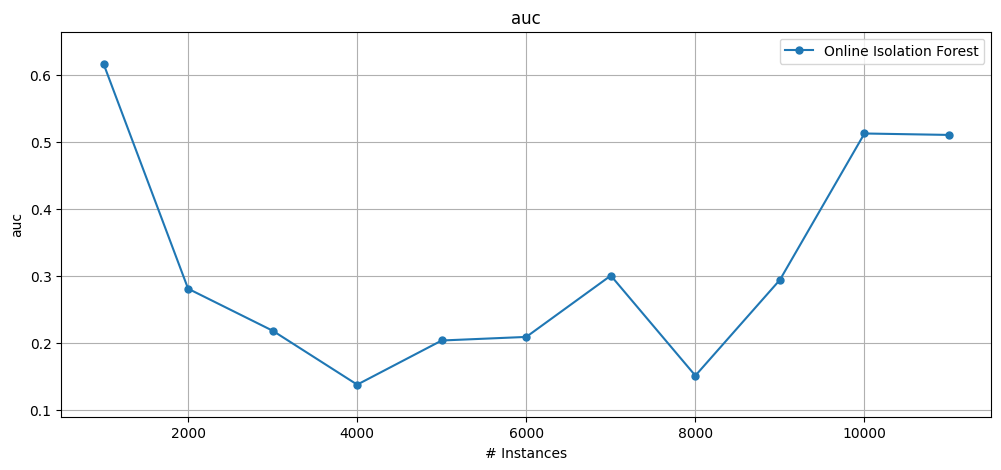
4 Comparing algorithms#
[6]:
plot_windowed_results(
results_hst, results_ae, results_oif, metric="auc", save_only=False
)
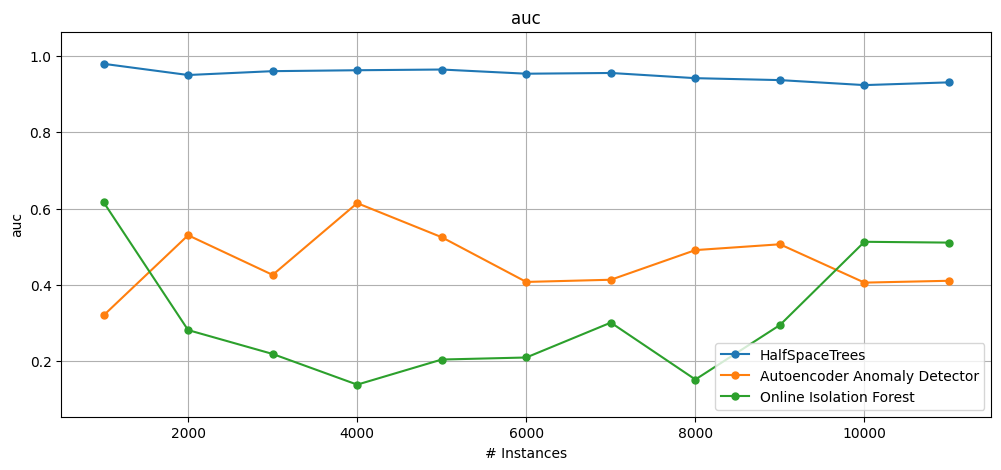