Clustering tutorial#
This tutorial demonstrates the experimental clustering API for capymoa. Clustering data streams refers to grouping data points into clusters as the data continuously flows in, which normally includes two phases:
Online Step
Micro-Cluster Formation: Incoming data points are incrementally processed and assigned to micro-clusters. Micro-clusters are small, temporary clusters that capture local density information and are typically represented by statistical summaries like centroid, weight, and radius.
Micro-Cluster Maintenance: The micro-clusters are periodically updated as new data arrives. This includes adjusting the micro-cluster centroids and merging or splitting clusters based on defined thresholds.
Offline Step: Periodically or upon request, micro-clusters are aggregated into macro-clusters (or simply clusters) to provide a higher-level view of the data.
This is an experimental API might change significantly in the near future.
More information about CapyMOA can be found in https://www.capymoa.org
last update on 31/07/2024
1. Creating and using a clusterer#
Example using
CluStream
andkMeans
for the offline stepThere is not evaluation included in the example below, just updating and plotting the cluster
The data is generated using
RandomRBFGeneratorDrift
We use a visualization function to print the clustering state
[1]:
from capymoa.clusterers import Clustream_with_kmeans as WithKmeans
from capymoa.stream.generator import RandomRBFGeneratorDrift
from capymoa.evaluation.visualization import plot_clustering_state
stream = RandomRBFGeneratorDrift(
number_of_attributes=2,
number_of_centroids=5,
number_of_drifting_centroids=1,
magnitude_of_change=0.001,
)
clustream = WithKmeans(
schema=stream.get_schema(),
time_window=100,
max_num_kernels=50,
kernel_radi_factor=2,
k_option=5,
)
[2]:
instancesSeen = 0
updateInterval = 100
while stream.has_more_instances() and instancesSeen < 300:
instance = stream.next_instance()
clustream.train(instance)
instancesSeen += 1
if instancesSeen % updateInterval == 0:
print(f"Processed {instancesSeen} instances.")
plot_clustering_state(clustream)
# by default, plot_clustering_state only shows the image and does not save it
Processed 100 instances.
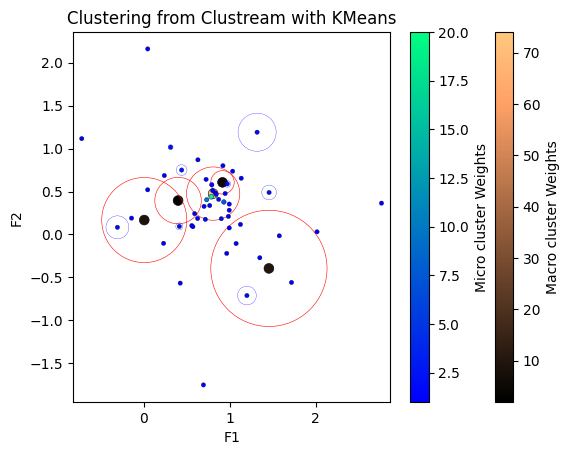
Processed 200 instances.
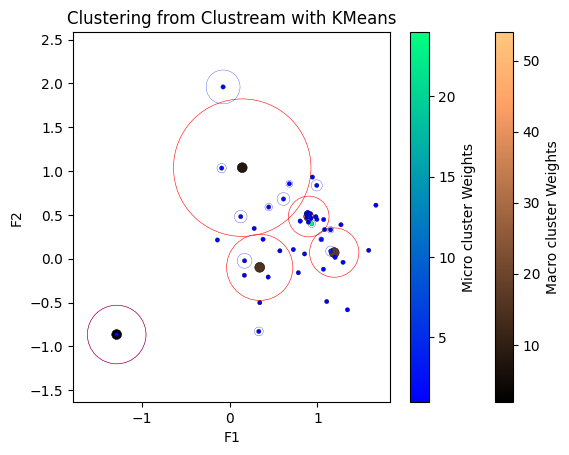
Processed 300 instances.
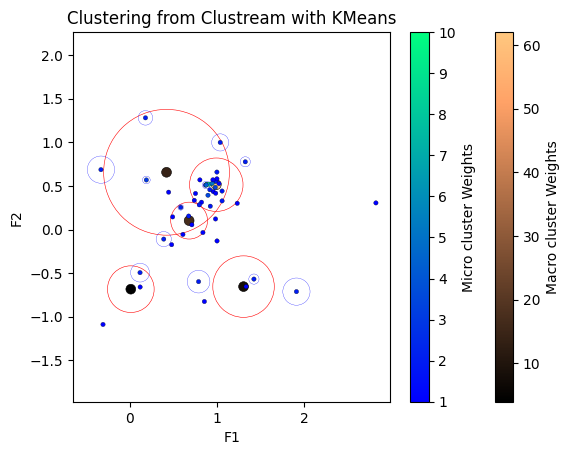
2. Using the Clustering Evaluator#
[3]:
from capymoa.evaluation import ClusteringEvaluator
[4]:
stream = RandomRBFGeneratorDrift(
number_of_attributes=2,
number_of_centroids=10,
number_of_drifting_centroids=1,
magnitude_of_change=0.001,
)
clustream = WithKmeans(
schema=stream.get_schema(),
time_window=1000,
max_num_kernels=25,
kernel_radi_factor=2,
k_option=5,
)
evaluator = ClusteringEvaluator(update_interval=100)
2.1 Plot the clustering state on demand#
[5]:
while stream.has_more_instances() and evaluator.get_instances_seen() < 1000:
instance = stream.next_instance()
clustream.train(instance)
evaluator.update(clustream)
instancesSeen = evaluator.get_instances_seen()
# purposefully arbitrary number
if instancesSeen == 157:
# can also skip show and only save
print(
f"Processed {instancesSeen} instances. Saving the figure without showing it."
)
plot_clustering_state(
clustream, show_fig=False, save_fig=True, figure_name="save_fig_dont_show"
)
Processed 157 instances. Saving the figure without showing it.
2.2 Plot the clustering evolution (gif)#
Passing
clean_up=False
to theplot_clustering_evolution
function will keep the intermediate figures used to create the gif.You need the
ClusteringEvaluator
to generate the gif.Default filename will be
<clusterer_name>_clustering_evolution.gif
[6]:
from capymoa.evaluation.visualization import plot_clustering_evolution
plot_clustering_evolution(evaluator, clean_up=True, frame_duration=1000)
[7]:
from IPython.display import Image
# Display the GIF
Image(filename="./Clustream_with_Kmeans_clustering_evolution.gif")
[7]:
<IPython.core.display.Image object>
3. Using DenStream with DBSCAN#
[8]:
from capymoa.clusterers import Denstream_with_dbscan as Denstream
denstream = Denstream(
schema=stream.get_schema(),
horizon=1000,
epsilon=0.04,
beta=0.2,
mu=1.2,
init_points=100,
offline_option=3,
lambda_option=0.25,
speed=200,
)
stream = RandomRBFGeneratorDrift(
number_of_attributes=2,
number_of_centroids=10,
number_of_drifting_centroids=1,
magnitude_of_change=0.001,
)
evaluator = ClusteringEvaluator(update_interval=100)
[9]:
while stream.has_more_instances() and evaluator.get_instances_seen() < 1000:
instance = stream.next_instance()
denstream.train(instance)
evaluator.update(denstream)
instancesSeen = evaluator.get_instances_seen()
You can choose the name of the gif file using the
filename
option
[10]:
# This will save the clusterer as output
plot_clustering_evolution(
evaluator, clean_up=True, filename="DeNSTReaM_clustering_custom_name.gif"
)
# Display the GIF
Image(filename="./DeNSTReaM_clustering_custom_name.gif")
[10]:
<IPython.core.display.Image object>
4. Using Clustream without macro-clustering#
[11]:
from capymoa.clusterers import Clustream as ClustreamMicro
clustream_micro = ClustreamMicro(
schema=stream.get_schema(),
kernel_radi_factor=2,
time_window=1000,
max_num_kernels=25,
)
stream = RandomRBFGeneratorDrift(
number_of_attributes=2,
number_of_centroids=10,
number_of_drifting_centroids=1,
magnitude_of_change=0.001,
)
evaluator = ClusteringEvaluator(update_interval=100)
[12]:
while stream.has_more_instances() and evaluator.get_instances_seen() < 1000:
instance = stream.next_instance()
clustream_micro.train(instance)
evaluator.update(clustream_micro)
instancesSeen = evaluator.get_instances_seen()
[13]:
# This will save the clusterer as output
plot_clustering_evolution(evaluator, clean_up=True)
# Display the GIF
Image(filename="./Clustream_clustering_evolution.gif")
[13]:
<IPython.core.display.Image object>
5. Using ClusTree#
[14]:
from capymoa.clusterers import ClusTree
clustree = ClusTree(
schema=stream.get_schema(), horizon=500, max_height=7, breadth_first_strategy=True
)
stream = RandomRBFGeneratorDrift(
number_of_attributes=2,
number_of_centroids=10,
number_of_drifting_centroids=1,
magnitude_of_change=0.001,
)
evaluator = ClusteringEvaluator(update_interval=100)
[15]:
while stream.has_more_instances() and evaluator.get_instances_seen() < 1000:
instance = stream.next_instance()
clustree.train(instance)
evaluator.update(clustree)
instancesSeen = evaluator.get_instances_seen()
[16]:
# This will save the clusterer as output
plot_clustering_evolution(evaluator, clean_up=True)
# Display the GIF
Image(filename="./ClusTree_clustering_evolution.gif")
[16]:
<IPython.core.display.Image object>