Drift Detection in CapyMOA#
In this tutorial, we show how to conduct drift detection using CapyMOA
Then test different drift detectors
Example using ADWIN
Evaluating detectors based on known drift location
More information about CapyMOA can be found in https://www.capymoa.org
last update on 25/07/2024
[1]:
import numpy as np
import pandas as pd
import capymoa.drift.detectors as detectors
Basic example#
Creating dummy data
[2]:
data_stream = np.random.randint(2, size=2000)
for i in range(999, 2000):
data_stream[i] = np.random.randint(6, high=12)
Basic drift detection example
[3]:
all_detectors = detectors.__all__
n_detections = {k: 0 for k in all_detectors}
for detector_name in all_detectors:
detector = getattr(detectors, detector_name)()
for i in range(2000):
detector.add_element(float(data_stream[i]))
if detector.detected_change():
n_detections[detector_name] += 1
print(pd.Series(n_detections))
ADWIN 2
CUSUM 1
DDM 1
EWMAChart 1
GeometricMovingAverage 1
HDDMAverage 126
HDDMWeighted 89
PageHinkley 1
RDDM 2
SEED 2
STEPD 1
ABCD 1
dtype: int64
Example using ADWIN#
[4]:
# detector = ADWIN(delta=0.001)
for i in range(2000):
detector.add_element(data_stream[i])
if detector.detected_change():
print(
"Change detected in data: " + str(data_stream[i]) + " - at index: " + str(i)
)
Change detected in data: 1 - at index: 24
Change detected in data: 6 - at index: 1010
[5]:
# Detection indices
detector.detection_index
[5]:
[1011, 2025, 3011]
[6]:
# Warning indices
detector.warning_index
[6]:
[1009, 1010, 2022, 2023, 2024, 3009, 3010]
[7]:
# Instance counter
detector.idx
[7]:
4000
Evaluating drift detectors#
Assuming the drift locations are known, you can evaluate detectors using EvaluateDetector class
This class takes a parameter called max_delay, which is the maximum number of instances for which we consider a detector to have detected a change. After max_delay instances, we assume that the change is obvious and have been missed by the detector.
[8]:
from capymoa.drift.eval_detector import EvaluateDetector
[9]:
eval = EvaluateDetector(max_delay=200)
The EvaluateDetector class takes two arguments for evaluating detectors:
The locations of the drift
The locations of the detections
[10]:
trues = np.array([1000])
preds = detector.detection_index
eval.calc_performance(preds, trues)
[10]:
mean_time_to_detect 11.0
missed_detection_ratio 0.0
mean_time_btw_false_alarms NaN
no_alarms_per_episode 0.0
dtype: float64
Multivariate Drift Detection#
[11]:
from capymoa.drift.detectors import ABCD
from capymoa.datasets import ElectricityTiny
detector = ABCD()
## Opening a file as a stream
stream = ElectricityTiny()
[12]:
i = 0
loss_values = []
while stream.has_more_instances and i < 5000:
i += 1
instance = stream.next_instance()
detector.add_element(instance)
loss_values.append(detector.loss())
if detector.detected_change():
print("Change detected at index: " + str(i))
Change detected at index: 2283
[13]:
import numpy as np
from capymoa.drift.detectors import ABCD
from capymoa.datasets import ElectricityTiny
detector = ABCD(model_id="pca")
## Opening a file as a stream
stream_change = np.hstack(
[np.random.uniform(0, 0.5, 3000), np.random.uniform(0.5, 1.0, 3000)]
)
stream_nochange = np.random.uniform(0, 1.0, len(stream_change))
stream = np.vstack([stream_change, stream_nochange]).T
print(f"A {stream.shape[-1]}-dimensional stream")
A 2-dimensional stream
[14]:
i = 0
loss_values = []
while i < len(stream):
instance = stream[i]
i += 1
detector.add_element(instance)
loss_values.append(detector.loss())
if detector.detected_change():
print("Change detected at index: " + str(i))
Change detected at index: 3063
[15]:
import matplotlib.pyplot as plt
import pandas as pd
plt.plot(pd.Series(loss_values).rolling(10).mean())
plt.title("ABCD with PCA")
plt.xlabel("# Instances")
plt.ylabel("Reconstruction loss")
[15]:
Text(0, 0.5, 'Reconstruction loss')
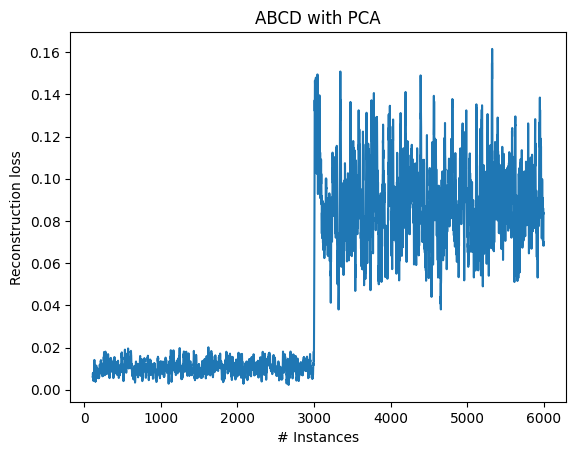
We see that a value of 1 as maximum reconstruction error is very conservative. By decreasing the maximum_absolute_value
parameter, we can make change detection faster as it makes the applied statistical test more sensitive.
[16]:
detector = ABCD(model_id="pca", maximum_absolute_value=0.3)
i = 0
loss_values = []
while i < len(stream):
instance = stream[i]
i += 1
detector.add_element(instance)
loss_values.append(detector.loss())
if detector.detected_change():
print("Change detected at index: " + str(i))
Change detected at index: 3024