8. Prediction Intervals for data streams#
How to utilise the prediction interval on regression tasks in CapyMOA
Two methods for obtaining prediction intervals are currently available in CapyMOA: MVE and AdaPI
More details about prediction intervals for streaming data can be found in the AdaPI paper:
More information about CapyMOA can be found in https://www.capymoa.org
notebook last updated on 01/08/2024
[1]:
from capymoa.datasets import Fried
# load data
fried_stream = Fried()
1. Basic prediction interval learner build-up#
An example of the use case of prediction interval in CapyMOA
Current available prediction interval learners require a regressive base model to work
[2]:
from capymoa.regressor import SOKNL
from capymoa.prediction_interval import MVE
# build prediction interval learner in regular manner
soknl = SOKNL(schema=fried_stream.get_schema(), ensemble_size=10)
mve = MVE(schema=fried_stream.get_schema(), base_learner=soknl)
# build prediction interval learner in in-line manner
mve_inline = MVE(
schema=fried_stream.get_schema(),
base_learner=SOKNL(schema=fried_stream.get_schema(), ensemble_size=10),
)
2. Creating evaluators#
We involve two types of prediction interval evaluators so far: basic (cumulative) and windowed
[3]:
from capymoa.evaluation.evaluation import (
PredictionIntervalEvaluator,
PredictionIntervalWindowedEvaluator,
)
# build prediction interval (basic and windowed) evaluators
mve_evaluator = PredictionIntervalEvaluator(schema=fried_stream.get_schema())
mve_windowed_evaluator = PredictionIntervalWindowedEvaluator(
schema=fried_stream.get_schema(), window_size=1000
)
3. Running test-then-train/prequential tasks manually#
don’t forget to train the models (call .train() function) at the end!
[4]:
# run test-then-train/prequential tasks
while fried_stream.has_more_instances():
instance = fried_stream.next_instance()
prediction = mve.predict(instance)
mve_evaluator.update(instance.y_value, prediction)
mve_windowed_evaluator.update(instance.y_value, prediction)
mve.train(instance)
4. Results from both evaluators#
[5]:
# show results
print(
f"MVE basic evaluation:\ncoverage: {mve_evaluator.coverage()}, NMPIW: {mve_evaluator.nmpiw()}"
)
print(
f"MVE windowed evaluation in last window:\ncoverage: {mve_windowed_evaluator.coverage()}, \nNMPIW: {mve_windowed_evaluator.nmpiw()}"
)
MVE basic evaluation:
coverage: 97.28, NMPIW: 30.66
MVE windowed evaluation in last window:
coverage: [98.9, 98.1, 96.7, 97.7, 97.4, 97.7, 98.0, 97.8, 97.2, 96.8, 97.2, 97.9, 97.1, 97.5, 98.2, 97.8, 96.4, 97.4, 96.6, 97.3, 98.7, 96.4, 97.2, 97.0, 97.4, 96.0, 97.5, 97.1, 96.8, 96.7, 97.7, 97.2, 98.0, 96.1, 97.5, 97.2, 96.9, 97.2, 96.9, 96.7],
NMPIW: [61.91, 46.44, 42.59, 43.82, 40.64, 40.08, 35.84, 38.32, 35.65, 39.25, 39.69, 36.09, 34.97, 34.95, 35.3, 34.79, 34.28, 33.46, 35.69, 35.64, 35.75, 35.03, 31.01, 33.91, 32.83, 33.54, 31.92, 31.31, 33.83, 32.07, 29.51, 33.25, 33.53, 31.93, 33.22, 29.3, 30.48, 29.39, 33.0, 32.14]
5. Wrap things up with prequential evaluation#
Prediction interval tasks also can be wrapped up with prequential evaluation in CapyMOA
[6]:
from capymoa.evaluation import prequential_evaluation
from capymoa.prediction_interval import AdaPI
# restart stream
fried_stream.restart()
# specify regressive model
regressive_learner = SOKNL(schema=fried_stream.get_schema(), ensemble_size=10)
# build prediction interval models
mve_learner = MVE(schema=fried_stream.get_schema(), base_learner=regressive_learner)
adapi_learner = AdaPI(
schema=fried_stream.get_schema(), base_learner=regressive_learner, limit=0.001
)
# gather results
mve_results = prequential_evaluation(
stream=fried_stream, learner=mve_learner, window_size=1000
)
adapi_results = prequential_evaluation(
stream=fried_stream, learner=adapi_learner, window_size=1000
)
# show overall results
print(
f"MVE coverage: {mve_results.cumulative.coverage()}, NMPIW: {mve_results.cumulative.nmpiw()}"
)
print(
f"AdaPI coverage: {adapi_results.cumulative.coverage()}, NMPIW: {adapi_results.cumulative.nmpiw()}"
)
MVE coverage: 97.28, NMPIW: 30.66
AdaPI coverage: 96.15, NMPIW: 28.53
6. Plots are also supported#
[7]:
from capymoa.evaluation.visualization import plot_windowed_results
# plot over time comparison
plot_windowed_results(mve_results, adapi_results, metric="coverage")
plot_windowed_results(mve_results, adapi_results, metric="nmpiw")
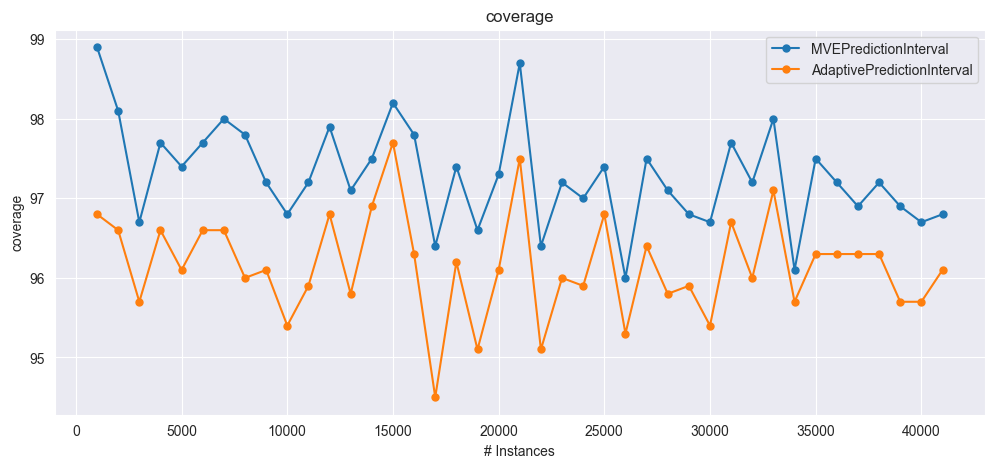
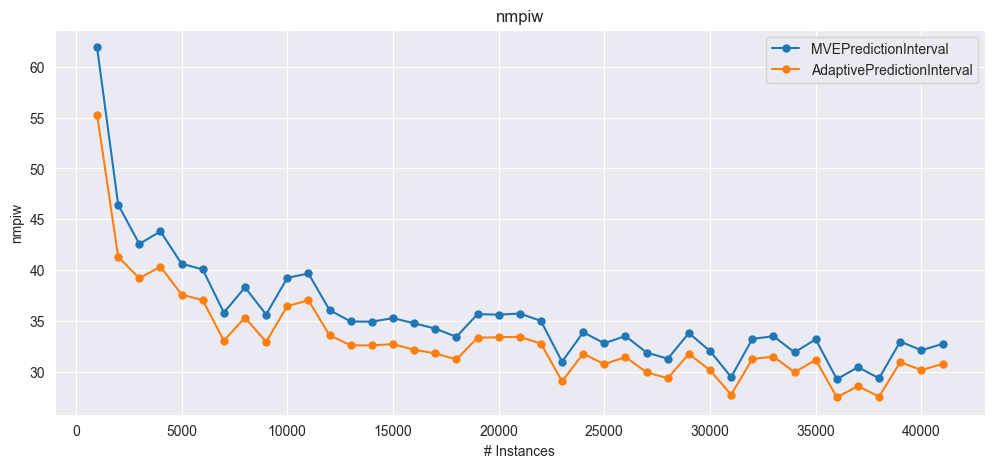
Plotting prediction intervals over time#
We also provide a visualization tool for plotting prediction intervals over time
The function
plot_prediction_interval
can be used to plot the prediction intervals over timeThe function can take one of two prediction interval results as input
Single result plotting example#
In order to plot the prediction interval over time, we need to have store the predictions and the ground truth values in the prediction interval results
The shaded area represents the prediction interval, while the solid line represents the regressive predictions.
The stared-markers represent the ground truth values that are covered by the intervals.
On the other hand, the cross-markers represent the ground truth values that are outside the intervals.
The colors can be adjusted by the
colors
parameter in the function as a list.start
andend
parameters can be used to specify the range of the plot.The
ground truth
andpredictions
can be omitted by setting theplot_truth
andplot_predictions
parameters toFalse
.
We have to set ``optimise`` to ``Flase`` to avoid subscribing problems
[8]:
new_mve_learner = MVE(
schema=fried_stream.get_schema(),
base_learner=SOKNL(schema=fried_stream.get_schema(), ensemble_size=10),
)
new_mve_results = prequential_evaluation(
stream=fried_stream,
learner=new_mve_learner,
window_size=1000,
optimise=False,
store_predictions=True,
store_y=True,
)
[9]:
from capymoa.evaluation.visualization import plot_prediction_interval
plot_prediction_interval(new_mve_results, start=300, end=500, colors=["coral"])
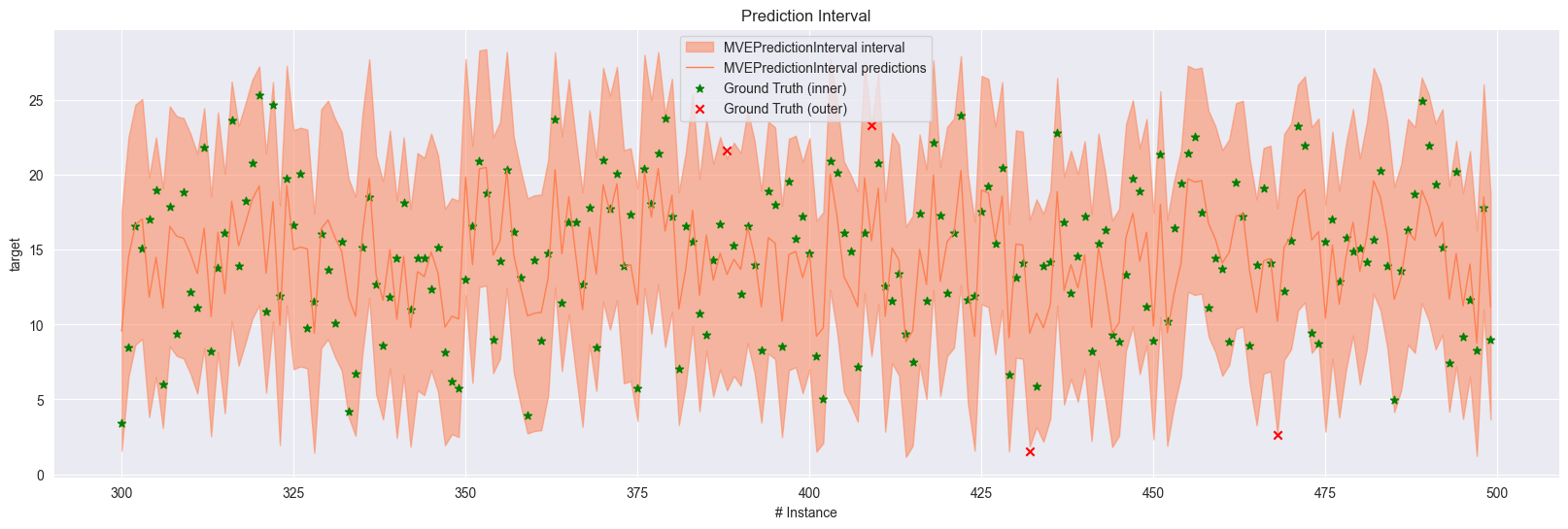
Two results plotting example#
For comparison purposes, we can also plot two prediction interval results over time.
We don’t take more results since it makes the plot too messy to read.
[10]:
# Let's add another results
new_adapi_learner = AdaPI(
schema=fried_stream.get_schema(),
base_learner=SOKNL(schema=fried_stream.get_schema(), ensemble_size=10),
limit=0.001,
)
new_adapi_results = prequential_evaluation(
stream=fried_stream,
learner=new_adapi_learner,
window_size=1000,
optimise=False,
store_predictions=True,
store_y=True,
)
[11]:
plot_prediction_interval(
new_mve_results,
new_adapi_results,
start=300,
end=500,
colors=["coral", "teal"],
plot_predictions=False,
)
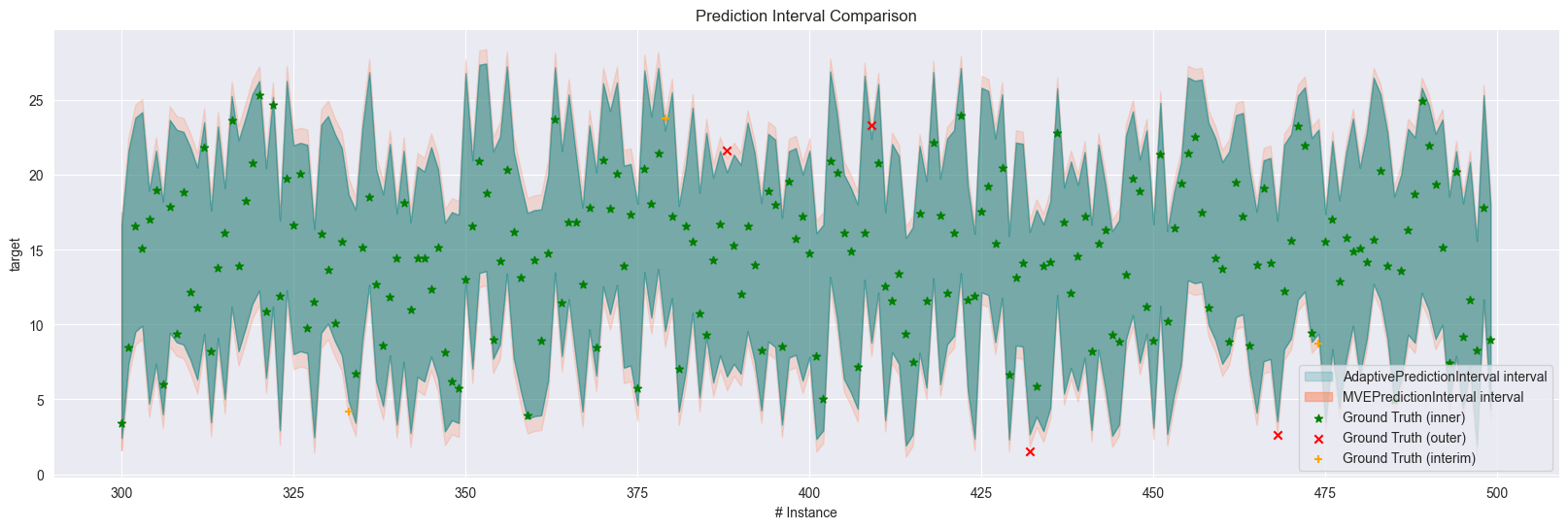
New “plus” markers represent the ground truth values that are covered by the narrower but not by wider intervals.
The function automatically puts the wider area to the back to make the narrower intervals more visible.